About
In the era of eHealth and personalized medicine, “big data” and “machine learning” are increasingly becoming part of the medical world. Algoritms are capable of supporting diagnostic and therapeutic processes, and offer added value for both healthcare professionals and patients. The field of big data, machine learning, deep learning and algorithm development and validation is often referred to as “data science”, and “data scientist” was mentioned in Harvard Business Review as “the sexiest job of the 21th century”. A commonly used visual representation of the field is Drew Conway’s Venn diagram (Figure [PK1] 1), that describes data science as a mix of content expertise, methodological knowledge, and IT skills.
Unfortunately, most healthcare professionals still consider the field of clinical data science as highly technical and something “for the IT whizzkids”. That leaves many interesting and valuable opportunies unexplored, and could even contribute to serious flaws in developed algorithms. Chen and Asch described machine learning’s “peak of inflated expectations” and suggest that “we can soften a subsequent crash into a ‘trough of disillusionment’ by fostering a stronger appreciation of the technology’s capabilities and limitations” [Chen, 2017]. They conclude that “combining machine-learning software with the best human clinician ‘hardware’ will permit delivery of care that out- performs what either can do alone.” We could not agree more.
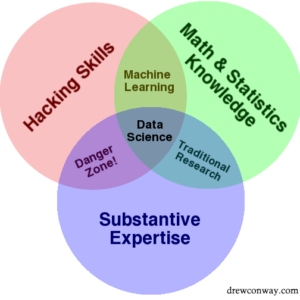
Data science Venn diagram by Drew Conway (reproduced with permission)
This book is for you, the healthcare professional and “best human clinician hardware” who would like to embrace the field of clinical data science, but who is still looking for a resource that explains the topic in non-engineering terminology. This book’s promise is “no math, no code”. It contains three sections that help you understand the transformation of data to information and to applications. It should be sufficient to give you a decent grasp on the topic for understanding and a solid foundation if you are to continue with active mastery of the field by taking programming courses online or in a classroom setting. Either way, we want you to get aboard.
Synopsis
This open access book comprehensively covers the fundamentals of clinical data science, focusing on data collection, modelling and clinical applications. Topics covered in the first section on data collection include: data sources, data at scale (big data), data stewardship (FAIR data) and related privacy concerns. Aspects of predictive modelling using techniques such as classification, regression or clustering, and prediction model validation will be covered in the second section. The third section covers aspects of (mobile) clinical decision support systems, operational excellence and value-based healthcare.
Thanks to
Our thanks go to the NFU Citrienfonds who made it financially possible to publish this ebook as open-access, to all authors for their valuable time and contributions, to Studio Piranha for the website, and to Springer Open for their help in the publishing process.